Labeling Tools for Machine Learning: A Comprehensive Guide
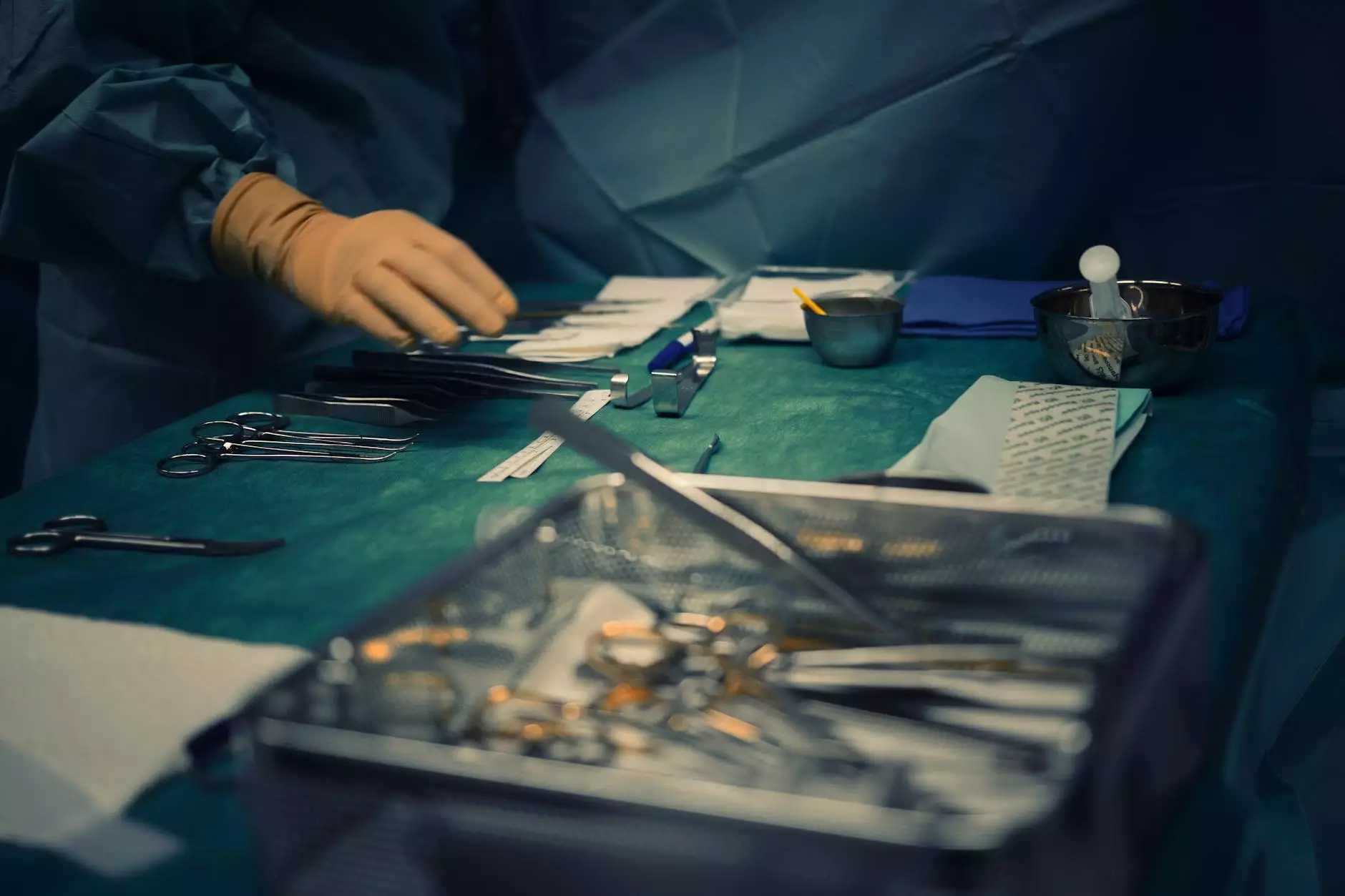
In the era of artificial intelligence (AI) and machine learning (ML), the significance of labeling tools for machine learning cannot be overstated. As organizations increasingly leverage data to train models, the need for precise and efficient data annotation becomes critical. This article delves into the intricate world of data annotation, exploring its techniques, tools, and the pivotal role it plays in machine learning workflows.
Understanding Data Annotation in Machine Learning
Data annotation is the process of labeling data to enable machine learning models to identify patterns, classify information, and make decisions. The quality and accuracy of labeling directly impact the performance of machine learning algorithms. Here are some key points to consider:
- Purpose of Data Annotation: The main goal is to provide a machine learning model with clear examples and labels so it can learn to recognize similar patterns in unannotated data.
- Types of Data: Data can include images, videos, audio, text, and various other formats, each requiring different labeling techniques.
- Human Oversight: Despite advances in AI, human input is often necessary to ensure that the annotations are accurate and relevant.
The Importance of Labeling Tools for Machine Learning
The demand for labeling tools has surged as businesses recognize the impact of high-quality labeled data on their machine learning projects. Here’s why labeling tools are essential:
- Improved Accuracy: Quality labeling tools reduce human error and enhance the accuracy of annotations, which is vital for the success of machine learning models.
- Efficiency Boost: Automated labeling tools can significantly decrease the time required for annotation, enabling faster development cycles and quicker deployment of AI solutions.
- Scalability: As data volumes increase, effective labeling tools allow organizations to scale their annotation processes without compromising quality.
Types of Labeling Tools for Machine Learning
Labeling tools can vary based on functionality, user experience, and specific use cases. Below are some overarching categories:
1. Image Annotation Tools
These tools focus on annotating images and can include functionalities such as:
- Bounding Boxes: Used to identify specific objects within an image.
- Segmentation: Detailed labeling of each pixel in an image, providing deeper insights.
- Landmark Annotation: Useful for facial recognition applications.
2. Text Annotation Tools
Text annotation tools help in identifying and labeling elements within text data, which can be crucial for natural language processing (NLP). Key features include:
- Entity Recognition: Identifying entities such as names, organizations, and locations.
- Sentiment Analysis: Labeling text based on sentiments expressed.
- Part-of-Speech Tagging: Labeling words with their corresponding part of speech.
3. Audio and Video Annotation Tools
These tools are designed for annotating audio clips and video frames, which includes capabilities like:
- Speech Transcription: Converting audio recordings into text.
- Frame Extraction: Labeling specific frames within a video for object tracking.
- Speaker Identification: Marking different speakers in an audio clip.
4. Hybrid Annotation Tools
Many modern labeling tools integrate features for various data types, enabling comprehensive annotation for diverse projects. This flexibility is particularly useful in multi-modal datasets.
How to Choose the Right Labeling Tool for Machine Learning
Selecting the right labeling tool is crucial for maximizing the quality of your machine learning models. Here are several factors to consider:
- User Interface (UI): A straightforward and intuitive UI can significantly enhance the productivity of annotators.
- Collaboration Features: Look for tools that allow multiple users to collaborate seamlessly, especially for large teams.
- Integration Capabilities: Choose a tool that easily integrates with your data workflow or machine learning pipelines.
- Support and Training: Comprehensive support and training ensure your team can effectively use the tool.
The Role of KeyLabs.ai in Data Annotation
At KeyLabs.ai, we understand the challenges associated with data annotation. Our suite of labeling tools for machine learning is designed to streamline the annotation process while enhancing accuracy and efficiency. Here are some distinctive features of our platform:
- Advanced Automation: Our tools leverage AI to assist human annotators, improving speed and reducing errors.
- Multi-format Compatibility: Our platform supports various data formats, catering to multiple machine learning needs.
- Real-time Collaboration: Teams can work together in real time, enhancing productivity and ensuring consistency.
- Analytics and Reporting: Comprehensive analytics allow organizations to measure the quality of annotations and improve processes.
The Future of Labeling Tools for Machine Learning
As machine learning continues to evolve, so do the tools and techniques used for data annotation. Emerging trends include:
- AI-Powered Annotation: More sophisticated algorithms will continue to enhance automation in the annotation process.
- Active Learning: This technique will allow models to identify and request additional annotations for uncertain data, creating a self-improving system.
- Community-Driven Annotations: Platforms that crowdsource annotations can lead to diverse datasets that reduce bias.
Conclusion
In conclusion, the success of machine learning projects hinges significantly on the quality of data annotations. Investing in robust labeling tools for machine learning is crucial for organizations aiming to harness the full potential of AI. With KeyLabs.ai’s innovative solutions, companies can streamline their data annotation processes, ensuring greater accuracy, efficiency, and scalability. As we look to the future, embracing advancements in AI-powered annotation and active learning will be vital for sustaining the momentum of machine learning innovations.
To learn more about our Data Annotation Tools and Data Annotation Platform, visit KeyLabs.ai today!